What is The Impact of Data Science on Businesses?
- AI/ML
- March 22, 2024
Data science holds immense transformative power when strategically employed by businesses to drive growth. This blog post offers an insightful overview of what data science is for businesses, its key components, its importance for businesses, future trends, and more.
In today’s fast-paced world, data is equal to wealth. Every click, swipe, and transaction generates a wealth of data and is like a treasure trove waiting to be unlocked. But what does this mean for businesses? How can they make sense of this vast sea of information?
Well, data science refers to extracting meaningful patterns and trends from vast datasets, helping businesses make informed decisions and gain a competitive edge in today’s dynamic marketplace.
In this blog, we will take you through the practical implications of data science across various industries, from retail and finance to healthcare and beyond. We will showcase how data science and its adjacent technologies can be leveraged to drive growth, streamline operations, and enhance customer experiences.
What is Data Science for Business?
Data science in business employs various data analysis techniques and tools to solve complex problems and drive decision-making within the business context. It involves collecting, processing, and analyzing large volumes of data to extract valuable insights and inform strategic actions that lead to improved efficiency, productivity, and profitability.
Data science best practices encompass several key components:
Data Collection: Gathering data from various sources, including customer interactions, transactions, social media, and IoT devices.
Data Cleaning and Preparation: Preparing the collected data for analysis by removing inconsistencies, errors, and irrelevant information.
Data Analysis: Utilizing statistical techniques, machine learning algorithms, and data visualization tools to identify patterns, trends, correlations, and anomalies within the data.
Insight Generation: Extracting actionable insights from the analyzed data that can be used to make informed business decisions and drive growth.
Predictive Modeling: Building predictive models to forecast future outcomes and trends, including customer behavior, market demand, and sales performance.
Optimization: Using data-driven insights to optimize business processes, marketing strategies, product offerings, and resource allocation for maximum efficiency and effectiveness.
Why is Data Science Important for Business?
According to the data of Precedence Research, the global market size for data science platforms soared to USD 112.12 billion in 2022. By 2032, it is projected to reach approximately USD 501.03 billion, marking a remarkable growth trajectory with a compound annual growth rate (CAGR) of 16.2% from 2023 to 2032. This growth is just the tip of the iceberg. In the near future, data science will help organizations make informed decisions, bring huge growth opportunities and benefits for businesses, and help them scale at larger levels. Here are some of those highlighted benefits:
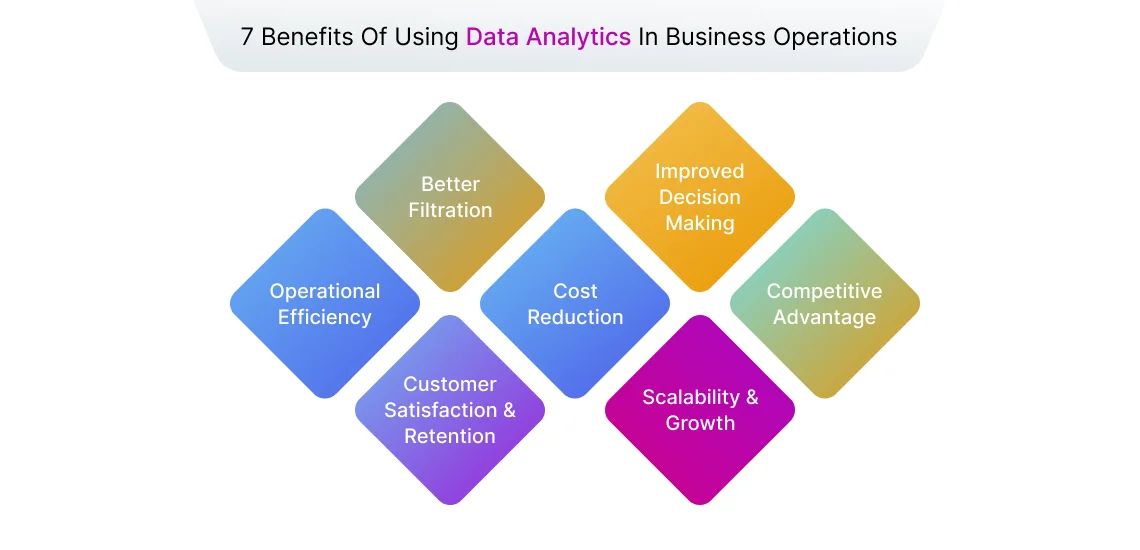
Better Filtration
Before analysis, data requires cleaning and preprocessing to remove inconsistencies, errors, or irrelevant information. Data science best practices automate the process, ensuring that only high-quality data is used for analysis or decision-making.
Furthermore, in datasets with many variables, Hire data scientists use feature selection and dimensionality reduction techniques to acknowledge the most relevant features or attributes. It reduces data complexity, eliminates anomalies, and improves data filtration.
Improved Decision Making
Data science in business helps organizations make wise decisions. The process uses data-driven insights and analysis to make strategic choices that are backed by empirical evidence and quantitative reasoning. The approach contrasts with traditional decision-making, which often relies on intuition, past experiences, or anecdotal evidence.
Operational Efficiency
Data science best practices like process mining, predictive maintenance, predictive analytics, and optimization algorithms help analyze the workflow within an organization. By identifying inefficiencies, bottlenecks, and areas for improvement, businesses can streamline their processes to eliminate unnecessary steps, reduce cycle times, and improve overall efficiency.
Cost Reduction
Data science supports businesses in optimizing resource allocation, streamlining operations, inventory optimization, reducing energy costs, predictive maintenance, fraud detection, etc. By analyzing historical data and using predictive modeling, businesses can generate profitability and reduce operation costs. The technology ensures the proper use of resources, manpower, materials, and equipment that minimize unnecessary expenditures.
Competitive Advantage
Data science in business helps organizations gain deeper insights into customer preferences, behavior, and needs. By leveraging this information, businesses can personalize products, services, and marketing efforts to better meet customer expectations, leading to higher user satisfaction and loyalty. This personalized approach can set businesses apart from competitors who offer generic, one-size-fits-all solutions.
Customer Satisfaction and Retention
Data science assists with collecting and integrating data from various sources, including transaction records, website interactions, social media activity, customer surveys, and demographic information. By aggregating and centralizing this data, businesses can create a comprehensive view of their customers across different touchpoints and channels.
After completing data collection, organizations can implement data science techniques like clustering algorithms to segment customers based on similarities in their behavior, preferences, and characteristics. This segmentation allows businesses to tailor their marketing messages, product offerings, and customer experiences to specific customer segments, increasing relevance and effectiveness.
Scalability and Growth
Data science techniques like AI and machine learning automate tasks and streamline workflows, enabling businesses to function more efficiently at scale without necessarily increasing the size of their workforce. It helps businesses to gain valuable insights into market trends, competitor behavior, and customer preferences. By staying informed about the competitive landscape, businesses can identify new growth opportunities and adapt their strategies accordingly.
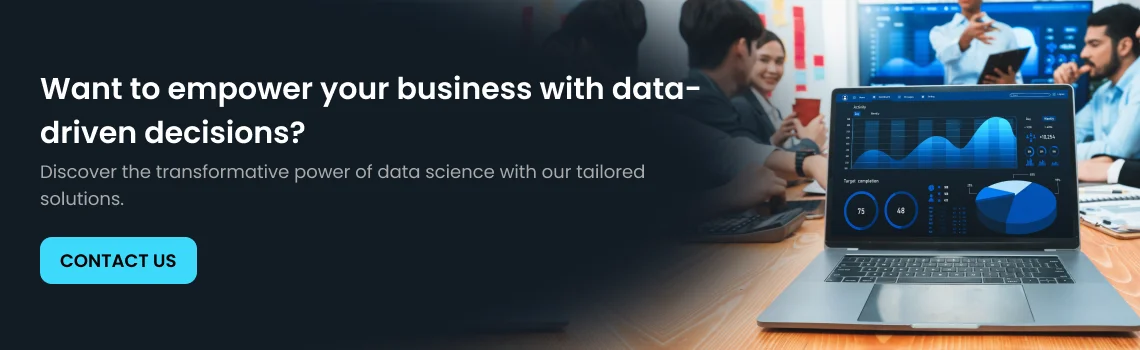
Future Trends Boosting the Use of Data Science in Business
From the integration of artificial intelligence and machine learning to the rise of edge computing and blockchain technology, businesses are using innovative approaches to extract actionable insights and drive decision-making. Let’s explore some of the key trends that will drive the adoption and impact of data science in businesses in the coming years.
Artificial Intelligence and Machine Learning
Artificial intelligence and machine learning algorithms help analyze large volumes of data to recognize patterns, trends, and relationships that may not be immediately apparent to human analysts. This analysis forms the foundation of data-driven decision-making in various domains.
Along with it, predictive analytics, recommendation systems, Natural Language Processing (NLP), image and video analysis, and anomaly detection enable businesses to implement advanced techniques to extract valuable insights from data, make better decisions, and drive innovation across various domains.
Also read: Top 12 AI Technology Trends for 2024
Edge Computing
Edge devices gather data from sensors, cameras, and other sources in their environment. It processes and filters the data locally to reduce the data volume transmitted to centralized servers, optimize bandwidth usage, and minimize latency. Moreover, edge computing platforms use data science techniques to analyze data locally and derive insights in real time.
Apart from these, edge computing facilitates the integration of data from multiple sources, including IoT sensors, social media feeds, and external databases, enabling comprehensive analysis and decision-making at the edge.
Also read: Cloud Security – The Detail Guide to Secure Cloud Computing
Blockchain for Data Security
Blockchain’s immutable ledger system ensures that once data is recorded in a block and added to the chain, it remains unalterable, safeguarding against tampering and unauthorized modifications. The decentralized network architecture further fortifies security by eliminating single points of failure and reducing vulnerability to malicious attacks.
Moreover, smart contracts enable automated, tamper-proof execution of transactions, enhancing security and compliance with predefined conditions. Blockchain offers transparent and auditable records of transactions, bolstering accountability and trust among participants.
Cross-domain Collaboration
Cross-domain collaboration connects experts from different domains, disciplines, and industries to collaborate on data-related projects and initiatives. Through collaboration, organizations can address complex challenges, uncover new insights, and drive innovation.
Data science teams may consist of individuals with backgrounds in data analysis, statistics, computer science, domain-specific knowledge, and business acumen, working together to analyze large datasets, develop predictive models, and derive actionable insights.
TinyML and Small Data
TinyML deploys machine learning models on resource-constrained devices, including microcontrollers, sensors, and IoT devices with limited processing power, memory, and energy resources. It enables ML algorithm implementation directly on edge devices, allowing for real-time inference and decision-making without relying on cloud-based services.
On the other hand, Small data are datasets with limited volume, velocity, or variety compared to big data. While big data typically involves massive datasets with complex structures, small data encompasses smaller-scale datasets that may be more manageable and accessible for analysis.
Conclusion
From personalized customer experiences to predictive maintenance and supply chain optimization, the applications of data science are limitless. By embracing data-driven decision-making, investing in the right tools and collaborating with a reliable digital transformation company, businesses can gain a competitive edge, adapt to changing market dynamics, and achieve sustainable growth in the digital age.
Why Choose MindInventory to Avail Data Science Services for Your Business?
MindInventory brings a unique blend of technical expertise and industry knowledge. We understand your business goals and tailor data science solutions that drive tangible results. Our experienced data scientists possess in-depth expertise in data analysis, machine learning, and AI to deliver tailored solutions that meet your specific business needs.
With a focus on innovation and continuous improvement, we stay abreast of the latest advancements in data science. Our experts work closely with your team every step of the way, from initial consultation to final implementation, ensuring alignment with your objectives and maximizing the impact of our solutions.
Determining if your business is ready for data science involves assessing several factors related to your organization’s goals, resources, and infrastructure. These factors include data availability and quality, technology infrastructure, analytical skills, organizational culture, business objectives, and resource allocation.
Businesses can collect and analyze a wide variety of data across different aspects of their operations. It includes customer data such as demographics, purchasing behavior, and interactions with products or services. Moreover, businesses can gather sales and transaction data, website and social media analytics, operational data related to inventory, supply chain, production processes, and financial data such as revenue, expenses, and profitability.
Businesses can tackle challenges by using data validation tools, implementing data governance policies, and training staff on data management best practices. Businesses should leverage technology to streamline data collection and analysis and automation tools to gather and process data more efficiently.