Generative AI in Healthcare: A Comprehensive Guide for Decision Makers
- AI/ML
- February 24, 2025
Similar to many other industries, generative AI in healthcare, too, plays a vital role. From medical research to drug discovery, disease diagnosis, medical imaging, and efficient administrative management with complete automation, it’s revolutionizing healthcare operations. This blog consists of an intensive analysis of the role of generative AI in healthcare, demystifying everything the decision-makers should be aware of.
Generative AI (artificial intelligence) uses AI to create new content such as text, images, videos, music, audio, and many more. Although it’s implemented across multiple industries, generative AI in healthcare is reshaping traditional healthcare operations. Babylon Health, Amazon Pharmacy, and Pfizer are among the top names leveraging Gen AI in healthcare settings.
While Babylon Health uses Gen AI for symptom-checking for accurate diagnostics and recommendations, Amazon Pharmacy integrates it to optimize medication recommendations. Similarly, Pfizer employs generative AI for drug discovery and clinical trials. From diagnosis to tailored medication and drug discovery, generative AI is reshaping the healthcare landscape completely.
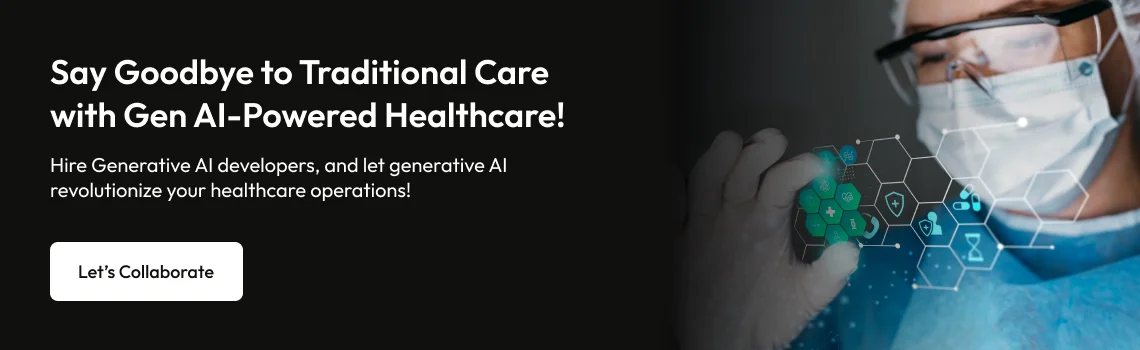
This blog explains everything about the role of generative AI in the healthcare industry, how it is transforming the same, and how decision-makers in healthcare could make the most out of this very technology. Let’s explore!
Generative AI In Healthcare: A Brief Introduction
Generative AI, a subset of AI in healthcare, is advancing medical treatment and patient experiences, transforming the healthcare ecosystem. With Gen AI, businesses create innovative solutions that enhance research, diagnosis, and treatment. These smart solutions produce synthetic medical data like realistic images to train diagnostic models; and design novel drug compounds, speeding up the drug discovery procedures.
Generative AI, in personalized medicine, customizes treatment plans aligning patients’ data, aiming to deliver treatment tailored to the very patient. It also helps create medical content, including clinical documentation and reports, mitigating administrative burdens.
Besides, Generative AI increases the ability of healthcare professionals by yielding new insights and optimizing clinical workflows, helping them with greater efficiency and precision in solutions. It paves the way for completely tailored, effective, and efficient healthcare solutions.
Generative AI In Healthcare Market Size And Uses Statistics
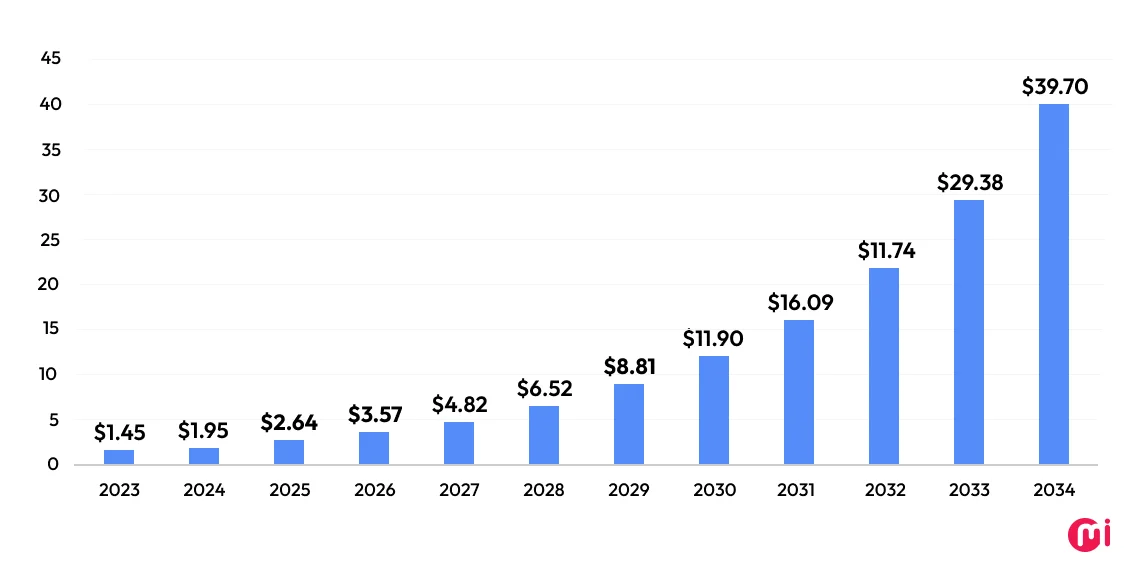
- Reports say that the size of the generative AI in the healthcare market is expected to reach $20.2 billion by 2032, exhibiting growth at a CAGR of 33.2% from 2024 to 2032.
- According to another study, the generative AI in healthcare market size globally is expected to be $2.67 billion in 2025 and is anticipated to reach $39.70 billion by 2034 at a CAGR of 35.17% between the forecast period (2024 to 2034).
- In the USA, the generative AI in the healthcare market is predicted to reach $10,495.69 million by 2034, at a CAGR of 35.45% from 2024 to 2034.
That’s how effulgent the present, and the future of Generative AI is in healthcare!
Applications of Generative AI In Healthcare with Real-Life Examples
When it comes to the role of generative AI in healthcare, it has a wide range of use cases. From automated administrative operations to medical imaging and diagnosis, medical research, drug discovery, and tailored medication, healthcare software development companies develop Gen AI-powered software solutions, taking healthcare to the next level.
Following are a few of the top-notch applications of generative AI in healthcare, along with real-life examples, for a clear yet intense learning experience:
1. Automated Administrative Operations
From smart appointment scheduling to automated transcription and updating EHR to automating claim processing and optimizing patient flow, Generative AI in healthcare automates administrative operations completely.
AI-enabled chatbots process patient inquiries and direct them to relevant departments and officials, and machine learning models help in coding and billing with optimal accuracy. It minimizes burdensome administrative tasks, lessens errors, and improves operational efficiency, enabling healthcare professionals to stay focused on patient care. Here’s how it does so:
- Appointment Scheduling: Gen AI-driven systems predict appointment times as per the patient’s need and preference, physician availability, and historical patterns, ensuring smart and automated appointment scheduling with reduced chances of cancellations.
- Updated Electronic Health Record (EHR): AI automatically updates EHRs to bring them in alignment with the medical histories, conversations, and lab results, ensuring records are accurate and updated without the need for human intervention.
- Automated Transcription: Generative AI transcribes doctor-patient interactions into excellently structured clinical notes, reducing the time taken to documentation.
- Automated Reminders: Generative AI in healthcare sends automated appointment reminders via SMS, email, or calls, reducing no-shows while improving clinical efficiency.
- Automated Billing: Generative AI efficiently reads through clinical documentation, services rendered, cross-references, and generates accurate billing codes, ensuring claims are submitted accurately and in compliance with the insurance requirements.
- Claim Processing Automation: Gen AI-powered systems automate the entire claims cycle, from generating claims forms to identifying missing data and even following up on rejected claims or underpayments.
- Automated Medical Coding: Generative AI helps medical professionals generate correct CPT, ICD-10, and HCPCS codes from clinical documentation, minimizing human errors and maximizing accuracy in coding.
- Insurance Eligibility Verification: Generative AI automatically verifies patients’ insurance eligibility, mitigating time spent on contacting insurers and improving the efficiency of patient registration.
- AI Chatbots: AI-powered chatbots tend to handle routine patient inquiries, such as questions about appointment scheduling, insurance coverage, basic medical queries, and billing, simplifying patient communication and support.
- Predictive Analytics for Patient Flow: Gen AI-powered systems predict admission rates, resource shortages, and potential bottlenecks, assisting healthcare professionals to make proactive decisions about the requirements.
Real-Life Example: Dragon Medical One, And Olive’s AI-Powered System
Nuance Communications’ Dragon Medical One is a real-life example of a generative AI-powered automated administrative operation in healthcare. It uses natural language processing (NLP) and generative AI and transcribes doctor-patient interactions in real time, turning speech into structured clinical documentation.
Besides, the technology helps with automated billing and coding by extracting relevant information from medical notes and patient records, ensuring accuracy and compliance. Automation lessens the time, effort, and errors, and increases efficiency and patient care as well as the overall workflow in a healthcare setting.
Olive’s AI-powered system is another example that automates back-office tasks such as revenue cycle management, patient scheduling, and claim processing. Furthermore, Olive efficiently handles complex administrative operations like claim review and authorization, report generation, and patient record operations, freeing employees to focus on core business activities.
2. Medical Imaging and Diagnostics
Medical Imaging is the process of creating visual representations of the human body that depict the internal structure of the human body utilized for clinical analysis, diagnosis, and treatment planning. They help recognize, track, and manage different medical ailments.
Generative AI is revolutionizing medical imaging and diagnostics, making them accessible, accurate, and efficient. Leveraging advanced machine learning and deep learning models, generative AI image interpretation, and diagnostic decision-making, allowing streamlined and more precise outcomes. Mentioned below are how Gen AI is easing medical imaging and diagnostics:
- Automated Image Interpretation: Generative AI algorithms automatically analyze medical images like CT scans, X-rays, MRIs, and ultrasounds, to identify abnormalities, lesions, tumors, and many other health conditions.
- Data Augmentation: Generative models like GANs (Generative Adversarial Networks), VAEs (Variational Autoencoders), or diffusion models, generate synthetic medical images, leveling up the available datasets to train machine learning models.
- Image Synthesis & Enhancement: Generative AI models such as GANs (Generative Adversarial Networks) and diffusion models synthesize high-quality medical images based on existing data that mitigates the need for additional scans, which is crucial from both the cost and patient safety perspective.
- Noise Reduction: Generative AI system reduces the noise and artifacts in medical images. It leads to more transparent and precise imaging.
- Predictive Diagnostic: Gen AI systems, trained on large datasets of mammograms, can detect and predict abnormalities, indicating the diseases in their early stages.
- Augmented Diagnosis and Decision Making: Generative AI-enabled systems detect and prioritize critical abnormalities in medical imaging, such as brain bleeds or spinal fractures, from CT scans. It enables radiologists to make more accurate diagnoses by flagging possible issues for early treatments.
Real-Life Example: Zebra Medical Vision
Zebra employs AI to analyze medical imaging, such as MRIs, X-rays, and CT scans, allowing an easy and efficient diagnostic of a variety of conditions like cardiovascular diseases, and neurological disorders. It automatically reads medical images, identifies abnormalities, and creates diagnostic reports for radiologists to review, enhancing accuracy and efficiency in diagnostic procedures.
3. Medical Research & Data Analysis
Generative AI-enabled medical research and data analysis is revolutionizing the healthcare ecosystem by accelerating discoveries, optimizing the way healthcare data is used, and improving clinical outcomes. The large amount of data generated in healthcare, such as clinical trials, patient records, imaging, and genomics are processed and assessed effectively in machine learning and deep learning intending to optimize the well-being of patients.
- Natural Language Processing (NLP): Generative AI, with NLP competencies, understands and interprets complex medical statutes, texts, and case law, enhancing the accuracy of extracting relevant information from broader and intricate healthcare documents.
- Efficient Data Processing: Generative AI analyzes a wider chunk of medical data, and automates data extraction and document review tasks. It results in mitigating time for administrative proceedings, freeing researchers to be focussed more on conducting other tasks.
- Search and Retrieval: Advanced search algorithms by Generative AI allow healthcare professionals to promptly retrieve the most relevant information, enhancing the efficiency of search and review of data.
- Medical Document Summarization: Generative AI summarizes larger medical documents, and provides a concise overview for researchers, allowing streamlined comprehensive decision-making, especially when it comes to dealing with extensive medical literature.
- Resource Optimization: By automating tasks and optimizing available resources, generative AI efficiently addresses resource constraints in medical research, especially benefiting those with limited funding.
- Predictive Analytics: Generative AI processes historical medical data, and offers insights into potential outcomes, enabling researchers for informed decision making.
Real-Life Example: Insilico Medicine
Insilico Medicine, a biotechnology company makes use of Generative AI for medical research and data analysis. It integrates advanced AI models like Generative Adversarial Networks (GANs) and Reinforcement Learning to accelerate the research of new drugs, analyze medical data, and uncover potential therapeutic pathways.
4. Drug Discovery and Development
Drug discovery and development consists of a multi-step process involving scientific research, experiments, and testing to bring new drugs into existence from a raw idea to market availability.
Due to lengthy research time, the complexity of biological systems, high failure rates, regulatory hurdles, and a huge amount of expense, drug discovery and development becomes complex. However, generative AI-enabled systems ease the hassles relating to drug discovery and development tasks.
- Data Integration and Analysis: Generative AI allows the integration and analysis of diverse data sources like electronic health records, omics data, and scientific literature, offering an extensive understanding of complex biological systems.
- Target Identification and Validation: Gen AI can assess biological datasets, identify potential drug targets, and validate their relevance in diseases, speeding up the process of drug discovery in the early stages.
- Compound Generation: Generative AI models are competent in proposing original chemical compounds with desired properties, allowing researchers to explore a wider chemical space for potential drugs.
- Biomarker Discovery: It’s a process of pinpointing biological indicators (biomarkers) to detect diseases, predict disease progression, track treatment response, or identify patients who would benefit from a specific therapy. Generative AI models, particularly Generative Adversarial Networks (GANs) and Variational Autoencoders (VAEs) are used to analyze vast amounts of biological data to generate new, relevant insights. These models are trained on large datasets like metabolomics data, genomic data, proteomics data, and clinical trial information.
- Clinical Trial Design: Gen AI models, such as Variational Autoencoders (VAEs), or Generative Adversarial Networks (GANs) can generate synthetic data by analyzing historical data from clinical trials intending to improve trial design, address target patient populations, and predict roadblocks. It boosts the overall drug development efficiency, ensuring optimized patient care.
- Repurposing Existing Drugs: Generative AI also predicts the effectiveness of existing drugs against diseases, hereby, identifying the new ways to use these drugs. It opens the doors for drug repurposing, expediting the development procedures.
Real-Life Example: Insilico Medicine
Insilico Medicine makes use of generative AI for drug discovery. It employs deep learning techniques to anticipate molecular behavior, ascertain potential drug consumers, and streamline the design of new therapeutic compounds. Insilico Medicine has discovered highly effective drugs for candidates affected by fibrosis and cancer.
5. Tailored Treatments
When it comes to tailored medication, doctors come across challenges in accurately interpreting vast genetic and molecular data. What’s more, integrating genetic information into traditional protocols, too, is complex and needs continuous learning to address the gaps in generic training. Thanks to the power of generative AI, it eases tailored medication. Here’s how!
- Data Analysis and Interpretation: Generative AI analyzes complex genetic and molecular data, enabling healthcare professionals to interpret relevant information for individual plans. It helps pinpoint generic and specific marker requirements and understand their implications for tailored treatment.
- Training & Education: Generative AI tends to contribute to educational initiatives by offering frequent updates on advancements in genomics and personalized medicine. It helps healthcare professionals stay in line with the latest technologies and treatment strategies.
- Clinical Decision Support: Generative AI provides healthcare organizations with immediate, evident-driven suggestions for customized treatment. It hugely improves the decision-making procedures by rapidly and accurately analyzing vast data.
- Ethical & Legal Compliance: The healthcare system, enabled by generative AI, powers a smooth navigation of ethical and legal considerations for tailored medication. It ensures that the AI systems adhere to privacy regulations and ethical standards to build patient trust and maintain compliance with healthcare laws.
- Pharmacogenomic Insights: Gen AI models are able to examine pharmacogenomic data intending to predict how individuals respond to medications. It helps customize drug prescriptions as per generic factors by incorporating pharmacogenomic information to improve treatment outcomes and mitigate adverse effects.
Real-Life Example: PathAI
PathAI makes use of Gen AI to create personalized plans, specifically in the field of pathology. The Generative AI models help PathAI to analyze tissue samples and pathology slides, offering oncologists intensive insights into cancer diagnoses and treatment strategies. It helps generate high-quality, synthetic images of pathology slides used to augment training data for AI models that detect cancerous cells, identify the aggressiveness of tumors, and find the subtle tissue patterns that may indicate early-stage cancer or other diseases.
6. Clinical Documentation and Medical Records
Generative AI has the competency to transform clinical documentation and medical record-keeping in healthcare organizations. Employing advanced machine learning models, such as deep learning and natural language processing (NLP), healthcare organizations can conveniently speed up documentation procedures and improve accuracy while elevating overall efficiency in medical workflows. Here’s how!
- Automated Clinical Documentation: Generative AI automatically creates clinical notes, dictations, and patient histories, eliminating the burden of manual tasks on clinicians. It does so with greater speed and excellence, enabling physicians to focus more on patient care as they are free from administrative tasks.
- Structured Data Entry with Improved Accuracy: Generative AI-powered systems help organize clinical data into structured formats that are easy to analyze. This involves turning free-text notes into standardized codes and formats including SNOMED CT, and ICD codes. These systems not only minimize human errors but also ensure essential details aren’t missed or misrepresented in medical records.
- Real-Time Decision Making: Generative AI analyzes patient data and clinical notes and helps real-time decision-making. It includes flagging potential risks, providing evidence-based recommendations, and suggesting relevant treatments based on the latest medical research.
- Enhanced Patient Engagement: Gen AI creates summaries of clinical records that are easy to understand, making it easier for patients to engage with their health data. These systems schedule appointments, send reminders, and provide educational content suitable to the patient records, allowing them to manage their health effortlessly.
Real-Life Example: 1upHealth
1upHealth employs Generative AI to accelerate its clinical documentation process and medical record management. The system integrates with Electronic Health Record (EHR) systems and applies Generative AI intending to automate the generation, interpretation, and updation of clinical documentation.
Using Generative AI, 1upHealth’s platform generates structured clinical documents based on unstructured patient data. For instance, after a doctor-patient consultation, the AI takes transcribed speech or written notes and turns them into standardized, and structured documentation like diagnoses, procedures, and medication prescriptions.
7. Telemedicine and Remote Patient Monitoring
Generative AI systems are enhancing telemedicine and remote patient monitoring (RPM) by improving the way healthcare services are delivered remotely. These systems are shaping the way healthcare service providers engage with patients, specifically as telemedicine and RPM are integrated into the mainstream healthcare system.
- Enhanced Virtual Consultation: Healthcare service providers can deploy gen AI-powered chatbots as triage tools to guide patients throughout initial symptom checks, offer health information, and even efficiently schedule virtual visits with doctors. These systems have competencies to simulate consultations for basic medical issues and offer tailored healthcare advice, ensuring streamlined operations of medications.
- Personalized Healthcare Monitoring with Remote Patient Monitoring (RPM): With the growing use of wearable devices, for example, smartwatches, glucose monitors, and ECG monitors, the generative AI analyzes large volumes of real-time health-related data gathered remotely. They process and interpret data from a variety of sources for example, vitals, sleep patterns, and physical activity to provide comprehensive, and tailored insights into a patient’s health.
- Chronic Disease Management: Chronic conditions like hypertension, diabetes, and asthma need frequent monitoring. Generative AI synthesizes data from RPM devices and patient records intending to track disease progression and offers tailored recommendations for suitable medication adjustment, lifestyle changes, and additional tests.
- Automated Documentation and Reporting: Generative AI automatically generates clinical notes from virtual consultations and RPM data helping clinicians save a significant amount of time. It also helps them document key elements of the consultation, such as diagnoses, treatment plans, and follow-up instructions, making sure to keep high-end records without adding to clinicians’ workload.
Real-Life Example: Babylon
One of the excellent examples of Generative AI in healthcare is Babylon. Babylon Health’s AI-powered telemedicine platform makes use of generative AI to analyze patient symptoms through natural language processing and offer virtual consultations with doctors. It integrates with wearable devices intending to monitor patients’ vitals remotely. The system flags health concerns, provides timely interventions, and delivers uninterrupted care for chronic conditions.
Why CEOs Should Invest In Generative AI Healthcare Solutions
The benefits of generative AI in healthcare include speed and accuracy in diagnoses, improved decision-making, reduced error, less expensive drug development and clinical trials, tailored medication, and many more. Here’s why CEOs should invest in Generative AI healthcare solutions:
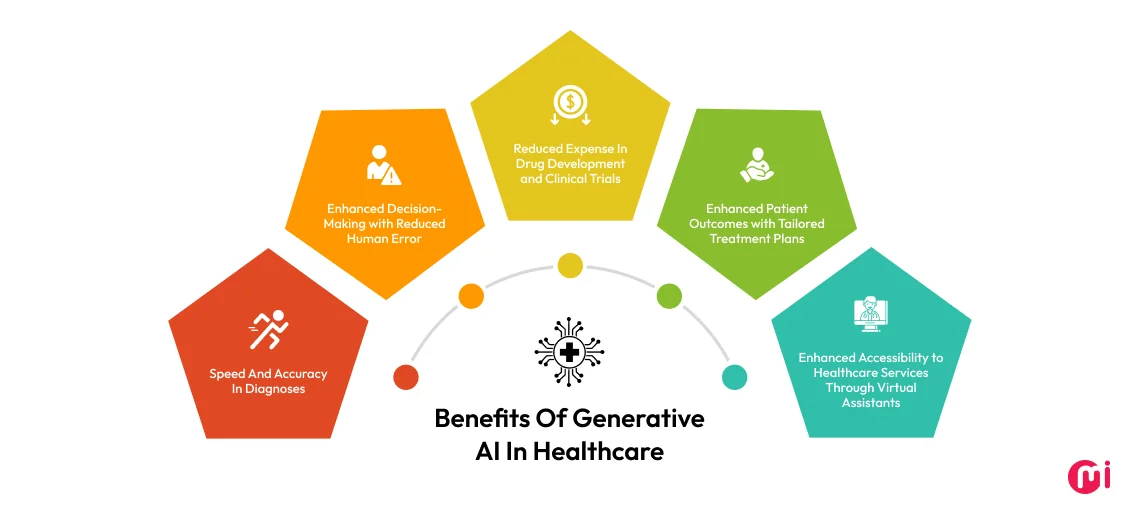
1. Speed And Accuracy In Diagnoses
Generative AI in healthcare quickly analyzes larger datasets, such as medical images, patient histories, and lab results, accelerating the complete diagnostic process. It identifies patterns that might otherwise be overlooked by humans, ensuring timely identification of health conditions, and leading to more effective and targeted healthcare offerings.
2. Data-Driven Decision-Making with Reduced Human Error
Generative AI processes vast amounts of medical data and provides evidence-based recommendations for healthcare. It enables clinicians to make informed decisions, resulting in limiting errors like misdiagnosis and inappropriate treatments. Limiting human biases and oversight, generative AI makes consistent and reliable medical choices for better patient care.
3. Reduced Expense In Drug Development and Clinical Trials
Generative AI in healthcare accelerates drug discovery by predicting molecular behavior and identifying potential drug candidates faster. What’s more, AI models also simulate clinical trial outcomes, limiting the need for lengthy and expensive trial phases. It speeds up the development of new treatments while lessening the research and testing costs.
4. Enhanced Patient Outcomes with Tailored Treatment Plans
AI in healthcare assesses genetic, medical, and environmental data and creates tailored treatment plans for patients. Anticipating how individual patients respond to different therapies, ensures effective and targeted treatments with different therapies, which ensures more effective and targeted treatments. This level of exactitude in medicine approach elevates patient outcomes while minimizing adverse effects and maximizing therapeutic benefits.
5. Excellent Healthcare Operation with Cost Effectiveness
Generative AI in healthcare automates various healthcare operations like clinical documentation, medical imaging, diagnostics, drug discovery, and more. Gen AI-enabled solutions automate repetitive tasks, optimizing resource allocation with no human intervention that contributes to reducing the cost of healthcare operations with enhanced efficiency and productivity.
6. Efficient Risk Management
Using generative AI in healthcare makes it possible to predict potential healthcare complications ahead of time. By doing so, GenAI helps develop preventive measures to overcome those issues. As generative AI excels in risk management, healthcare organizations don’t need to worry about risk management, freeing them to focus more on other essential activities.
7. Enhanced Accessibility to Healthcare Services Through Virtual Assistants
AI-powered virtual assistants provide 24/7 access to healthcare solutions, ensuring guidance, symptom checking, and appointment scheduling. It ensures enhanced accessibility, specifically for underserved or rural populations. Moreover, virtual assistants help with preventive care advice, medication reminders, and follow-up consultations, speeding up overall healthcare efficiency and accuracy.
Future of Generative AI In the Healthcare Ecosystem
From AI-powered medication to revolutionized drug discovery, and development, and enhanced hospital administration and workflow optimization, the generative AI in healthcare will be revolutionizing the future. Here’s how!
AI-Powered Advanced Medication
Analyzing genetic, environmental, and lifestyle data, generative AI will develop tailored treatment plans for precision medication. AI-powered algorithms will detect diseases like Alzheimer’s, cancer, and heart conditions at early stages, ensuring early disease detection. Besides, machine learning models will forecast patients’ health deterioration and circumvent difficulties through proactive intervention.
Revolutionized Drug Discovery & Development
AI will accelerate drug discovery by identifying promising compounds in a reduced amount of time and cost. Deep learning will help anticipate how molecules interact, thereby, improving drug formulation with mitigated failures. Gen AI will contribute to repurposing existing drugs by identifying new applications for them, hence, expediting treatment for major diseases.
Enhanced Medical Imaging and Robotics
AI-assisted radiology will reign supreme in the future. Gen AI in healthcare will enhance accuracy in MRIs, CT scans, and X-rays, helping radiologists to detect abnormalities. It also will ensure automated image analysis by quickly processing medical images, mitigating diagnostic turnaround time. Furthermore, Gen AI-powered robots will be helping in minimally invasive surgeries, maximizing precision and minimizing recovery times.
Hospital Administration & Workflow Optimization
Gen AI will be automating repetitive tasks like appointment scheduling, medical transcription, billing, and insurance claims in healthcare settings. Optimizing resource allocation, and improving hospital workflow and patient throughput, Gen AI-enabled solutions increase efficiency while reducing costs. Moreover, chatbots and voice assistants will be answering queries and schedule visits, thereby enhancing patient engagement.
Generative AI In Healthcare: Challenges & Solutions
Although there are benefits, implementing generative AI for healthcare comes with a few challenges as well. Following are the ways through which organizations can circumvent these challenges when AI is implemented in healthcare settings:
Collaboration with Healthcare Professionals
Promoting collaboration between data analysts, AI specialists, and medical professionals is challenging because of differing expertise and priorities. For example, clinicians focus on patient care, while AI specialists might lack clinical insights, and data analysts may not properly understand medical workflows. This disconnect is likely to lead to AI solutions that are difficult to integrate or fail to meet real-world needs.
Involving clinicians in the Gen AI enablement process in healthcare is a must-have that allows the development of a solution that is aligned with clinical workflow and addresses real-life challenges in healthcare.
Ethical Guidelines with Limiting Bias
Improper ethical guidelines and biases can cause AI models in healthcare to reinforce existing disparities, and lead to inaccurate diagnoses or unequal treatment recommendations. It might worsen healthcare inequalities, threaten patient outcomes, and erode trust in AI systems, eventually undermining the quality of care.
Therefore, setting up ethical guidelines for the use of AI in healthcare, and limiting biases in training data and algorithms ensures fair and unbiased outcomes, specifically in crucial areas such as diagnostics and treatment recommendations.
Data Privacy And Security Issues
Generative AI requires a humongous amount of patient data including genetic data, clinical data, medical images, etc. to train accurate models. AI models, if not secured properly, could be vulnerable to cyberattacks, leading to unauthorized access to sensitive healthcare information.
Businesses should use data encryption and decentralization; anonymization, and de-identification to prioritize robust data privacy to ensure compliance with healthcare privacy regulations.
Continuous Training And Education
Improper training and education for healthcare professionals on AI tools puts a risk of misuse or misinterpretation of AI outputs. Clinicians might struggle to understand AI recommendations, leading to poor decision-making, inconsistent patient care, and resistance to adoption, finally hindering the integration and effectiveness of AI in healthcare.
Hence, healthcare organizations should make sure that healthcare professionals receive frequent training and education to effectively comprehend and use AI tools, bringing them in line with the most recent advancements, ethical considerations, and excellent approaches in AI applications.
Clinical Validation & Regulatory Compliance
In the absence of proper clinical validation and adherence to regulatory compliance, AI tools are unlikely to be thoroughly tested for safety or effectiveness. This might lead to incorrect diagnoses, inappropriate treatments, or regulatory penalties.
Hence, it’s essential to ensure that generative AI models undergo thorough clinical testing and validation, ensuring optimal accuracy and reliability, adhering to regulatory compliance, and obtaining the needed approvals before AI implementation in a healthcare setting.
Integration And Scalability
With improper integration and scalability, AI tools might struggle to work seamlessly with existing healthcare systems. It can cause disruptions in workflow and lead to inefficient resource utilization, data inconsistencies, and sluggish adoption. In case of lack of scalability, AI solutions may fail to meet the requirements of larger or growing healthcare settings.
Therefore, it’s crucial to ensure an accurate integration with the existing healthcare systems when developing. The system should be compatible with different hospital IT systems and Electronic Health Records (EHR).
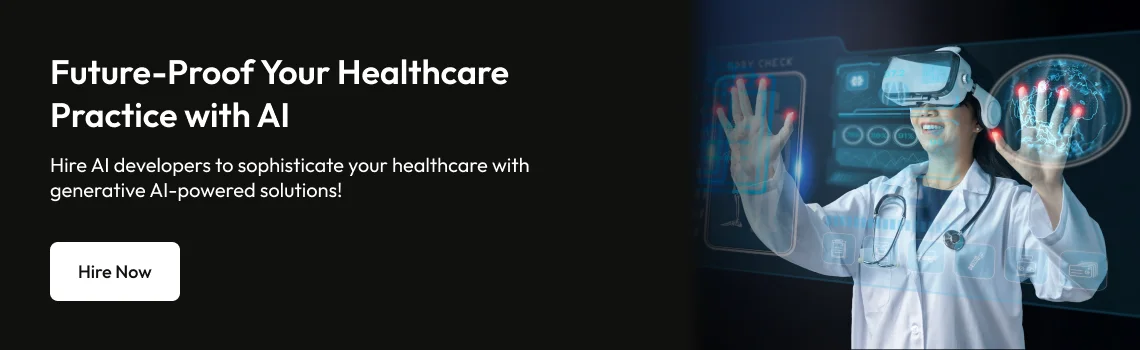
Harness the Power of Generative AI for Smarter Healthcare with MindInventory
MindInventory is a leading Generative AI development company, offering Gen AI development services to businesses of all sizes. Our generative AI developers harness the power of GANs, Transformers like ChatGPT; Open Source LLMs like Llama 2 and DALL-E, Text-to-Speech Avatar creation tools, Stable Diffusion, and more to deliver excellent solutions for healthcare organizations worldwide.
Sully.ai and Bipedai are among a few of the AI-powered software solutions built by our dexterous AI engineers. They allow users to leverage the power of AI and take their business to the next level.
Sully.ai is an AI-enabled solution that addresses the challenges, setting new standards for AI-driven healthcare automation. It resulted in a 56% improvement in overall efficiency, a 33% enhancement in prescription note generation, and a 27% optimization in the AI model’s performance.
Bipedai, a mobile app is designed specifically to complement the AI-enabled smart navigation harness. The client, implementing Bipedai, experienced an exceptional outcome, which is a 75% increase in obstacle avoidance efficiency, a 60% reduction in navigation-related stress, and a 50% acceleration in user satisfaction when it comes to GPS instructions.
Are you still relying on traditional healthcare operations? If yes, it’s to transition it into an AI-powered healthcare system to embrace an elevated healthcare experience for both patients and healthcare organizations. Get in touch with our expert to bring your ideas to reality and revolutionize the way of healthcare operations.
FAQs on Generative AI in Healthcare
Some use cases of generative AI in healthcare involve medical research, drug discovery, medical imaging, disease diagnosis, personalized treatment plans, administrative management, and more.
Leading companies include Insilico Medicine, Tempus, Atomwise, Zymergen, Babylon Health, Amazon Pharmacy, Pfizer, and others who are integrating AI to optimize clinical and operational outcomes.